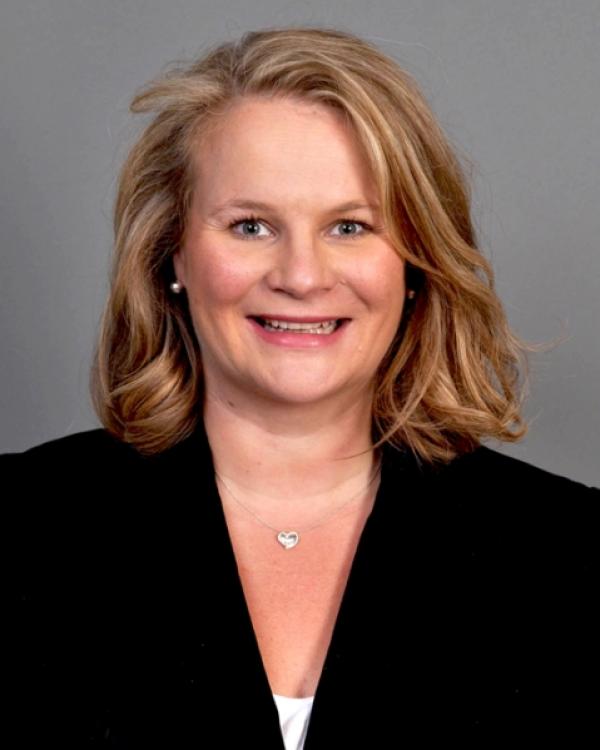
According to ResearchGate, an article co-authored by Karen Nylund-Gibson of the Department of Education at the Gevirtz School was the most cited publication from a UC Santa Barbara author in March 2016. Nylund and co-authors Tihomir Asparouhov and Bengt O. Muthén published “Deciding on the Number of Classes in Latent Class Analysis and Growth Mixture Modeling: A Monte Carlo Simulation Study” in the October 2007 issue of Structural Equation Modeling: A Multidisciplinary Journal. The article was cited over 1500 times in the month and is accessible on-line.
Mixture modeling is a widely applied data analysis technique used to identify unobserved heterogeneity in a population. Despite mixture models’ usefulness in practice, one unresolved issue in the application of mixture models is that there is not one commonly accepted statistical indicator for deciding on the number of classes in a study population. This article presents the results of a simulation study that examines the performance of likelihood-based tests and the traditionally used Information Criterion (ICs) used for determining the number of classes in mixture modeling. We look at the performance of these tests and indexes for 3 types of mixture models: latent class analysis (LCA), a factor mixture model (FMA), and a growth mixture models (GMM). We evaluate the ability of the tests and indexes to correctly identify the number of classes at three different sample sizes (n D 200, 500, 1,000). Whereas the Bayesian Information Criterion performed the best of the ICs, the bootstrap likelihood ratio test proved to be a very consistent indicator of classes across all of the models considered.
Karen Nylund-Gibson is an Associate Professor of quantitative research methodology in the Department of Education. She has been at UCSB since 2009. Prior to joining the department, she was a Postdoctoral Fellow at the Department of Mental Health at Johns Hopkins University. She earned her Ph.D. at UCLA, working with Bengt Muthén. Her research focus is on latent variable models, specifically mixture models and she has published many articles and book chapters on developments, best practices, and applications of latent class analysis, latent transition analysis, and growth mixture modeling.